Project Partners
Partner in Turkey: Istanbul Technical University, Embedded System Design Laboratory
Partner in Iran: Urmia University, Asst. Prof. Dr. Morteza Mousazadeh
Funding Organization: TÜBİTAK 2535 İran Bilim, Araştırma ve Teknoloji Bakanlığı (MSRT) ile İkili İşbirliği Programı
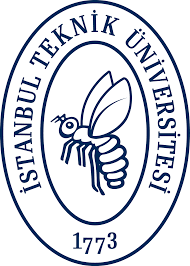
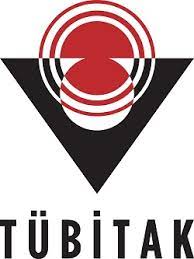
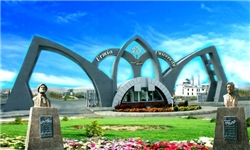
Project Team
Leader: Prof. Dr. Berna Örs Yalçın
Student: — Announcement
Project Summary
Embedded systems are developed specifically for the purpose of providing low-cost performance requirements such as speed, accuracy, and reliability. By definition, it contains application-specific hardware and software components. However, as the target system grows, the need for workforce and experience diversity for the design of the system increases. Therefore, the user control of the system becomes more difficult. It is very important that software support for hardware is provided in order to be able to use a system easily, to update it if necessary, and to be able to operate in accordance with other embedded systems. Many applications can be implemented with application specific inexpensive hardware units. However, the interest still is toward to general-purpose, expensive solutions designed to support different applications because of the lack of software development environment. On the other hand, the application areas of embedded systems can be extended by supporting the embedded systems with appropriate software and by developing efficient signal processing and decision-making algorithms specific to these systems. The increase of embedded systems where researchers and users can easily develop on them means that many applications can become cheap, practical, and capable of responding to changing conditions. At this point, it is necessary to follow a design process for designing an embedded system with all its software and hardware components. This necessity requires a combination of hardware, software and an environment for software development design in order to form a base for implementation of the specific application chosen in this project. Then, cost-sensitive application software design is the last requirement. Hence, the proposed project is interdisciplinary.
This project includes the design and implementation of a system on chip which includes an application specific instruction set processor and this processor’ peripherals. For the completion of the system on chip design we will deal with the following steps:
- Modeling the system for drive fatigue monitoring and verification of the model
- Implementation of RISC-V processor on an FPGA
- Realizing the model of the system for drive fatigue monitoring on the RISC-V processor implemented on the FPGA
- Extension of the instructions of RISC-V processor for drive fatigue monitoring and implementation on the FPGA
- Realizing the model of the system for drive fatigue monitoring on the RISC-V processor on FPGA by using the extended instruction set and system tests
- Implementation of the instruction set extended RISC-V processor as an ASIC
- Realizing the model of the system for drive fatigue monitoring on the ASIC of RISC-V processor by using the extended instruction set and system test
Following these methods, it was aimed to increase the project to THS5 level. Under the project, an application area that is considered to be important for Iran and Turkey have been selected.
Every year many car accidents occur around the world due to the driver’s fatigue or lack of concentration which results in many death and financial losses. The traffic accidents in Iran and Turkey is higher than the world average. In the field of driver support systems, a solution will be produced which includes machine learning and image processing techniques to solve two main issues of driver assist system 1) The fatigue measurement and 2) The concentration measurement. The solution will be a real time and inexpensive embedded system. The application, which is one of the outputs of the project, will help the drivers.
Application-oriented expansion of the processor will result in real-time and cost-effective implementation of this application, which includes image processing and machine learning. The fact that both hardware and software units will be designed for communication between the processor and peripherals will ensure that the system works in accordance with the embedded systems developed by different hardware manufacturers. From rough hardware to peripherals, application software and end user interface, the design process will be improved. Through this process, researchers will be able to design and implement their own systems that include special processors that provide different requirements for different applications. The final system on chip can be used in different applications with some software changes, hence it is scalable and updatable with only application specific software development.
Methodology
The processing unit will be designed to be able to access the peripherals such as DMA controller, MIPI CSI 2 (MIPI Camera Serial Interface 2), USB connection for data communication. In order to guarantee a healthy communication between the processor and the peripherals, the processor must be compatible with standard buses such as AXI4. AXI Bus can support many peripherals with the help of APB bridges. The standard buses are advantageous in hardware and software driver availability.
All the components will be integrated with a careful plan. First, these fundamental components will be designed and tested:
- Software model of DFD, involving image processing and machine learning algorithms.
- Essential RISC-V implementation on FPGA.
- Instruction extended RISC-V implementation on board.
Integration 1 – Rough hardware: Once B is completed, then integration of RISC-V with camera and porting all hardware components on development board can be started, before completing the DFD software model or Instruction extension. This way, any obstacles towards rough hardware integration will be detected and eliminated. The first integration is also required to collect data.
Integration 2 – Algorithm on real data: The data collected as a result of implementation B, will be used as an input to train DFD model in A. Therefore, the model itself will already be integrated with the real data acquired by the project setup. Accuracy of the model can be correctly determined with the real data.
Integration 3 – Processor and the software model: Final model parameters are used for instruction extension. Joint work between the algorithm design and instruction extension is required because, if the partner who implements the algorithm on RISC-V sees a problem on the results, the algorithmic partner should be contacted. This integration brings the algorithm implemented on RISC-V, for the first time in the project.
Integration 4 – Updated software model and the processor: After a list of new instructions are available, the DFD algorithm will be revisited and re-written using the new instructions. The compiler will as well be tested on this integration. With this integration the software flow from algorithm down to machine code through compiler will be examined.
Integration Ultimate – Complete system: The machine code generated by the compiler from the algorithm will burn the instruction-set extended RISC-V which is implemented on the FPGA of the development board with cameras and other peripherals.